AI vs Doctor: Lung Tumor Recognition on a Chest X-ray
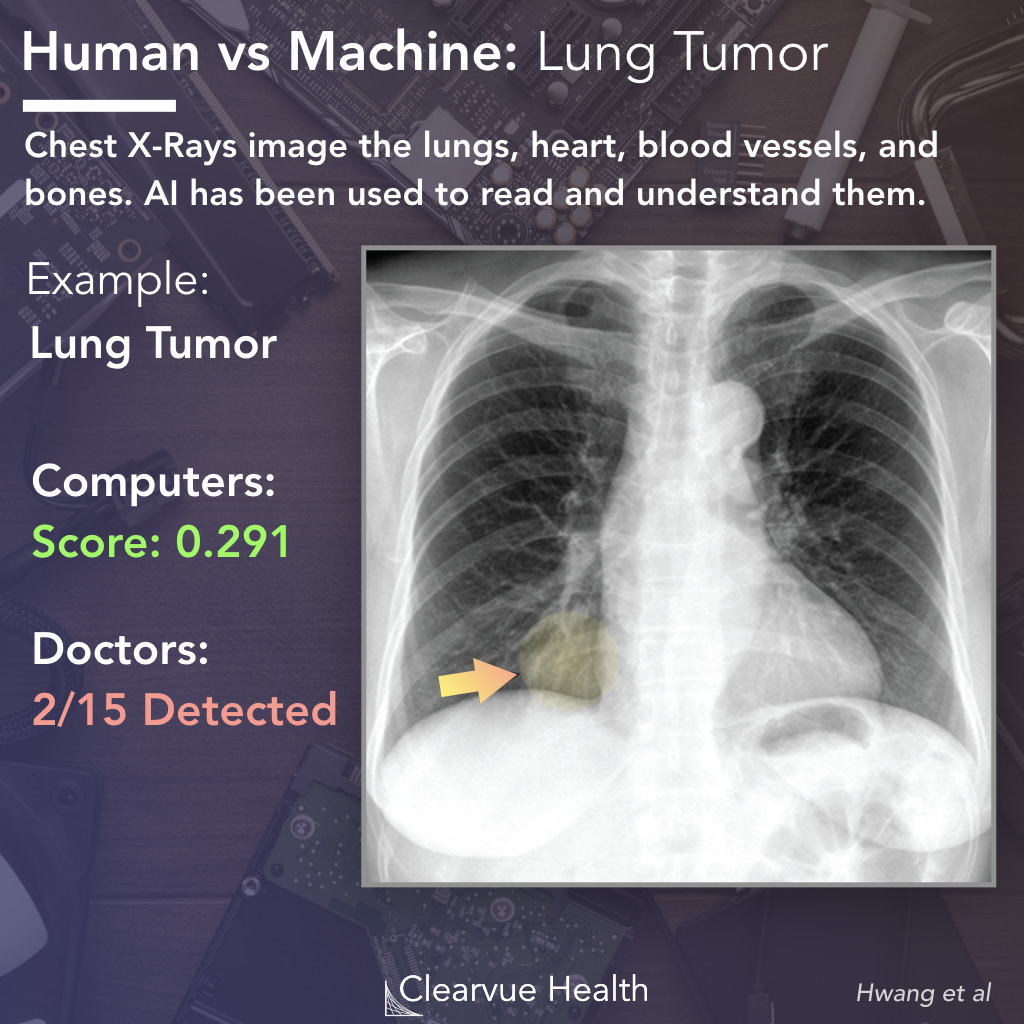
Figure 1: AI vs Doctor: Lung Tumor Recognition on a Chest X-ray. A Deep Learning Algorithm and 15 doctors were asked to read the above chest x-ray. The Deep Learning algorithm identified a possible tumor with a 0.291 probability score. The tumor was only identified by 2/15 doctors. The lung tumor is highlighted in yellow for presentation. Highlighting and the arrow was not present during the test.
Artificial Intelligence will eventually transform nearly every industry. Medicine is no exception. In particular, AI has gotten much better at reading images. Advances in parallel computing and deep learning have made near-human level image analysis possible.
In a recent paper, researchers tested an AI against 15 doctors of different levels of training to see how well an AI could read chest x-rays. Could it beat an average doctor? A Radiologist? A Specialist Radiologist?
The example above was one of the more challenging examples used. The patient in this case had a lung tumor (highlighted in yellow) that was particularly hard to read.
Only 2/15 doctors managed to identify the tumor. The AI was able to identify it and correctly locate it within the chest x-ray, with an albeit low probabiliy score of 0.291.
+
+
Validation Method - This study validated their AI on multiple datsets from external institutions. This reduces the possiblity that the AI learned based on features specific to one department or hospital.
+
Multiple Physician Categories Used - The validation method showed significant and expected differences between physicians and their training level, suggesting that the validation method was accurate.
+
Effect Size - This study showed a significant and clinically relevant effect. Large effect sizes are less likely due to statistical error or random chance alone.
-
Only tested in a lab setting - While the AI was evaluated among multiple datasets, this was not tested in a true clinical settings with real patients in real time.
Deep learning is a subset of machine learning that uses multiple layers of artifical neural networks. These networks use algorithms similar to the neurons of the human brain. Hundreds or thousands of parallel mathematical computations are used to analyze and classify data. They have been particularly effective in recognizing and understanding images. Common applications of deep learning include radiology, self-driving cars, and speech recognition.
Source: Development and Validation of a Deep Learning–Based Automated Detection Algorithm for Major Thoracic Diseases on Chest Radiographs
AI vs Doctor: Pneumonia Recognition on a Chest X-ray
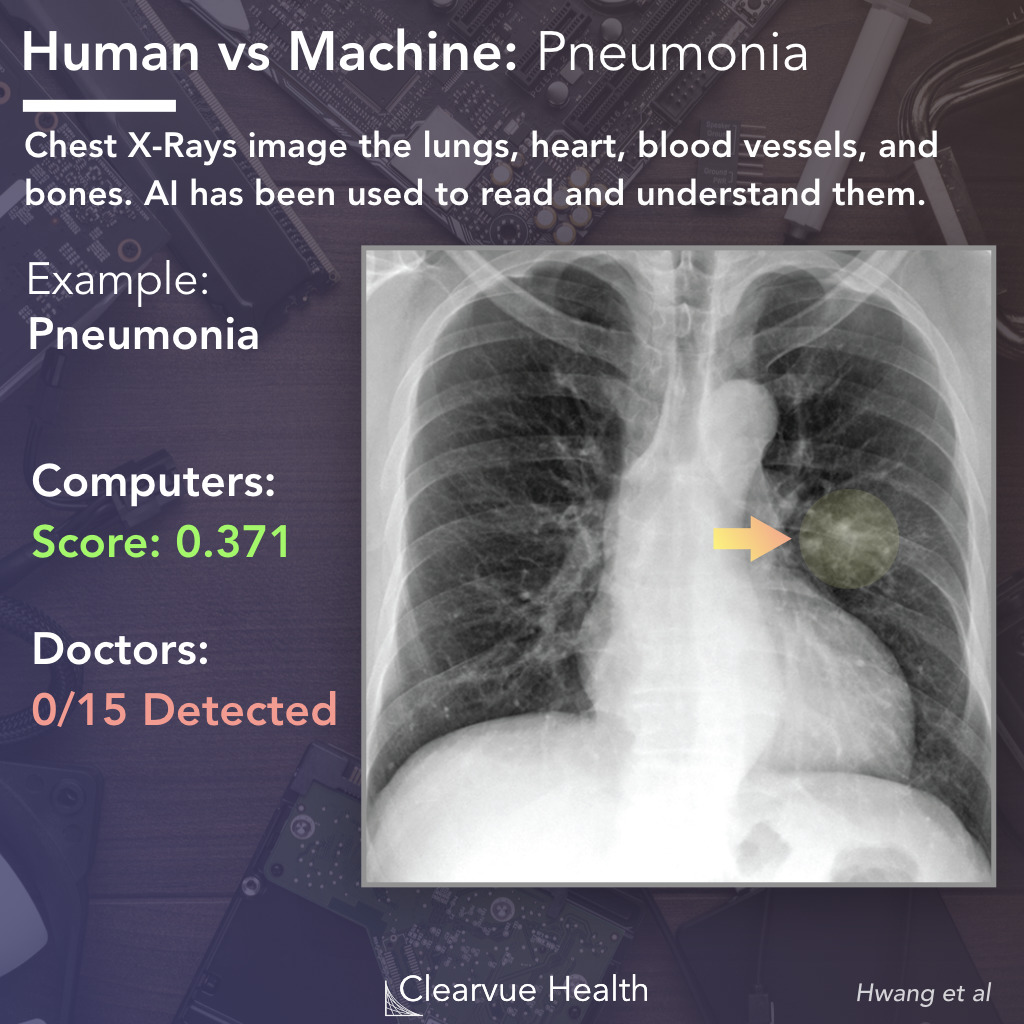
Figure 2: AI vs Doctor: Pneumonia Recognition on a Chest X-ray. A Deep Learning Algorithm and 15 doctors were asked to read the above chest x-ray. The Deep Learning algorithm identified a possible opacity from pneumonia with a 0.371 probability score. The pneumonia was missed by all 15 doctors. The lesion is highlighted in yellow for presentation. Highlighting and the arrow was not present during the test.
In a second representative example, the AI and doctors were asked to diagnose a patient based on the above chest x-ray. The X-ray contained what doctors call a "ground glass opacity." In this case, the opacity was from a case of pneumonia.
In this particular example, an AI was able to correctly locate this lesion with a probability score of 0.371, while all 15/15 doctors missed it.
Source: Development and Validation of a Deep Learning–Based Automated Detection Algorithm for Major Thoracic Diseases on Chest Radiographs
ROC Performance Evaluation for Doctors vs AI
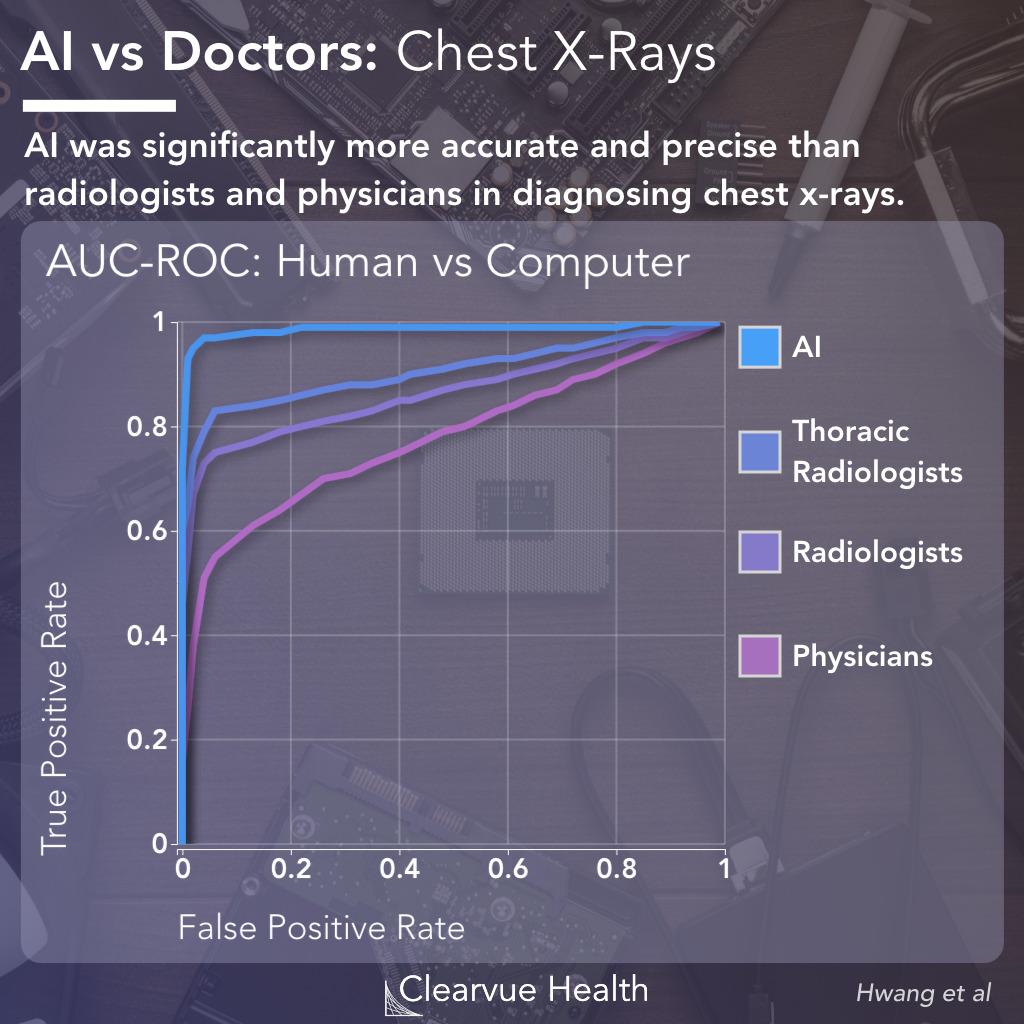
Figure 3: AUC Performance Evaluation for Doctors vs AI. AUC-ROC curves showed that the AI was significantly more accurate, with a higher true positive rate and a lower false positive rate, than doctors, including thoracic radiologists, radiologists, and physicians.
Across the entire dataset, the AI managed to detect images with higher sensitivity and specificity than doctors, as shown by the ROC curves above. The "higher" the curve, the more accurate the diagnosis.
DLAD had a AUCROC of 0.983, vs an AUCROC of 0.932 for specialist thoracic radiologists, 0.896 for board certified radiologists and 0.614 for nonradiology physicians.
An ROC curve measures how well a algorithm can distinguish or classify a dataset. It measures true positive rates against false positive rates. This provides one of the best characterizations of an algorithm's performance. For example, an algorithm that identifies a person as having cancer every single time will have a 100% true positive rate for cancer diagnosis, as every patient with cancer will be diagnosed. However, it will also have a 100% false positive rate as well as every patient without cancer will be diagnosed. The ROC curve allows us to see this relationship across different levels of sensitivity.
AI Assist with Chest X-Rays
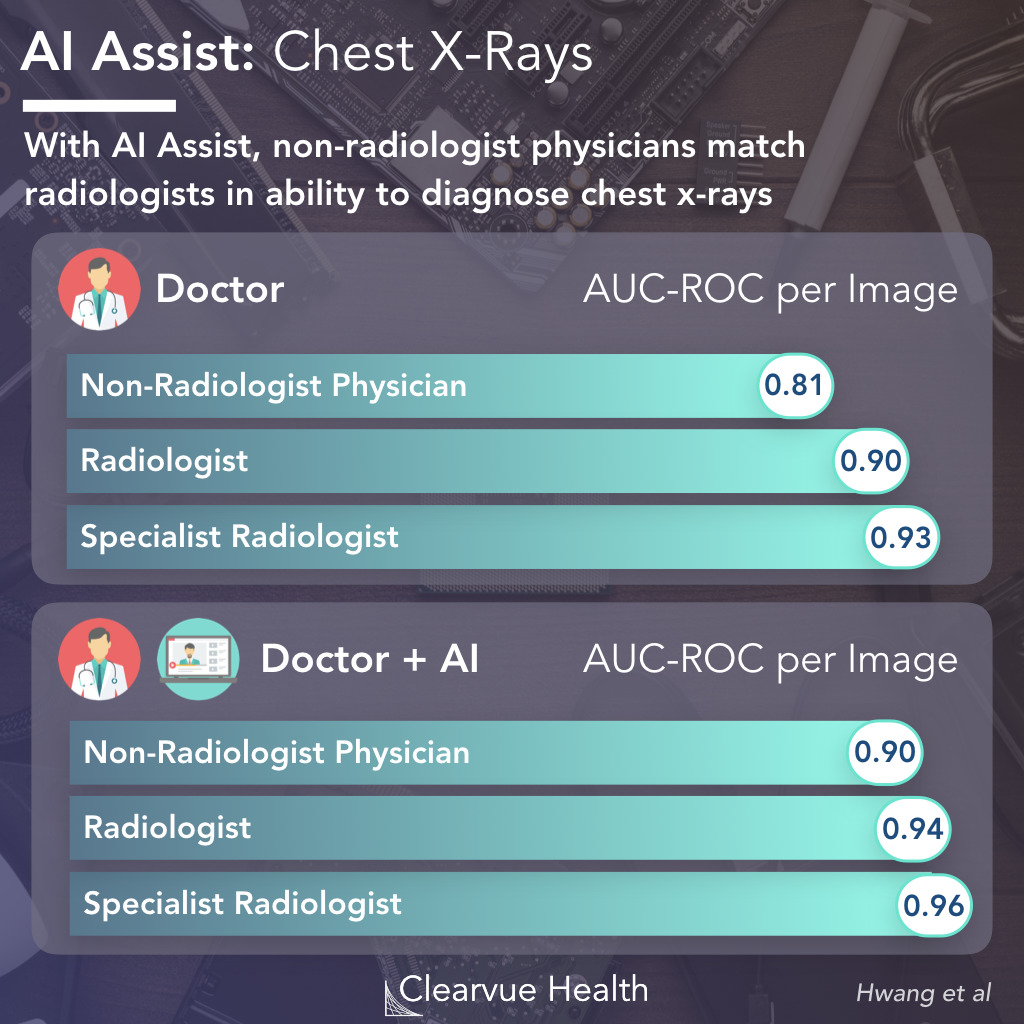
Figure 4: AI Assist with Chest X-Rays. Doctors were evaluated alone and with the assistance of an AI. With AI, doctors were more accurate in their diagnosis. Non-radiologist physicians read chest x-rays with approximately the safe accuracy as radiologists when assisted with an AI.
Initially, AI will likely be used to assist doctors in diagnosis. Researchers found that non-radiologists who had the assistance of an AI were able to diagnose chest x-rays as well as radiologists without an AI. Doctors of all levels of training benefited from having an AI assist in their diagnosis.
Key Takeaways
These data suggest an exciting future for medicine when it comes to AI. Well trained algorithms have the potential to improve diagnosis of all types. Patients receive chest x-rays all the time in hospitals. If algorithms can help doctors be more accurate in their diagnosis, we can potentially pick up more cases of cancer and other diseases earlier before they spread.